Research project: Multi-parameter Persistent Homology |
---|
This project is funded by the Austrian Science Fund (FWF) under grant P 33765-N (2021-25).
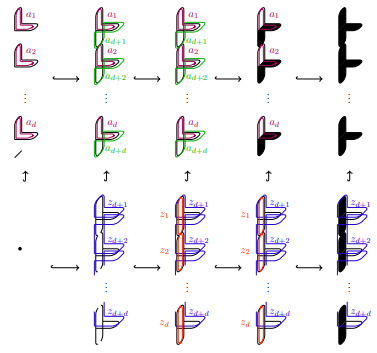
Topological data analysis is a vibrant research discipline from applied topology that combines representation theory, homological algebra, algorithms, and data analysis. Its success stems from the combination of sound mathematical theory, rich application areas and fast algorithms. The field of multi-parameter persistent homology extends the concept by analyzing data sets with several real scale parameters. The algorithmic aspects of the theory, however, have been rarely studied in the community until very recently. This is partially due to the fact that existing solutions for the single-parameter case do not extend easily to the multi-parameter case.
For multi-parameter persistence, we want to establish an algorithmic layer to facilitate the transfer from theoretical work into application areas.
This includes fundamental mathematical questions, directed towards simplification and computability, the design of algorithmic solutions with provable theoretical guarantees, and the publication of software to make our findings useful in application areas.
The list of publications below also includes work performed before the runtime of the grant.
Publications |
---|
- Michael Kerber, Alexander Rolle: Fast Minimal Presentations of Bi-Graded Persistence Modules. SIAM Symposium on Algorithm Engineering and Experiments (ALENEX 2021). Available at arXiv:2010.15623.
- Michael Kerber: Multi-Parameter Persistent Homology is Practical. Extended abstract for the workshop Topological Data Analysis and Beyond within the 34th Conference on Neural Information Processing Systems. (NeurIPS 2020) (poster).
- Mickael Buchet, Emerson G. Escolar: Every 1D Persistence Module is a Restriction of Some Indecomposable 2D Persistence Module. Journal of Applied and Computational Topology, 2020 (link).
- Michael Kerber, Arnur Nigmetov: Efficient Approximation of the Matching Distance for 2-parameter persistence. Proceedings of the 36th International Symposium on Computational Geometry (SoCG 2020), pp. 53:1-53:16. Available at arXiv:1912.05826.
- Havard Bjerkevik, Magnus Botnan, Michael Kerber: Computing the interleaving distance is NP-hard. Foundations of Computational Mathematics 20, pp.1237-1271, 2020. (link).
- Rene Corbet, Ulderico Fugacci, Michael Kerber, Claudia Landi, Bei Wang: A Kernel for Multi-Parameter Persistent Homology. Computer and Graphics X (2), 2019. Special Section on SMI 2019 (link) (Best paper award).
- Michael Kerber, Michael Lesnick, Steve Oudot: Exact computation of the matching distance on 2-parameter persistence modules. Proceedings of the 35th International Symposium on Computational Geometry (SoCG 2019), pp. 46:1-46:15. Available at arXiv:1812.09085.
- Ulderico Fugacci, Michael Kerber: Chunk Reduction for Multi-Parameter Persistent Homology. Proceedings of the 35th International Symposium on Computational Geometry (SoCG 2019), pp.37:1-37:14. Available at arXiv:1812.08580.
- Mickael Buchet, Emerson Escolar: Realizations of Indecomposable Persistence Modules of Arbitrarily Large Dimension. Proceedings of the 34th International Symposium on Computational Geometry (SoCG 2018), pp.15:1-15:13. Available at arXiv:1803.05722.
- Rene Corbet, Michael Kerber: The Representation Theorem of Persistent Homology Revisited and Generalized. Journal of Applied and Computational Topology (link) Available at arXiv:1707.08864.